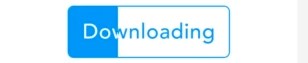

Imagine that in an identity verification scenario, the observed short-time-lapse FNMR is 1% when the application is initially fielded.

Furthermore, when they restricted their analysis to a subset of higher-quality images, they found an even larger increase in the FNMR. This corresponds to an increase in the FNMR of slightly over 150%. 5 They found that the FNMR went from 2.5% for short-term matches (less than six months) to 6.7% for long-term matches (over two years). The longer time interval consistently rejects authentic matches at a higher rate over a wide range of decision threshold scores.Īnother study by researchers from Clarkson University and West Virginia University showed a similar result. The false non-match rate for iris images taken one month apart (‘short’) and three years apart (‘long’). 4 The data plotted in Figure 2 shows that the FNMR is consistently higher for long time-lapse compared to short time-lapse across the range of decision threshold values.įigure 2. For our three-year data set, using the VeriEye iris matcher, and with a decision threshold that corresponds to a one in two million false match rate, we observed a 150% increase in the FNMR. However, our results did show a change in the authentic distribution, resulting in an increase in the false non-match rate (FNMR), i.e., the fraction of authentic matches that are rejected.Įstimating the magnitude of the increase in the FNMR is difficult, since it varies based on the value of the decision threshold, the matching algorithm used, and other factors. Our results did not show any change in the impostor distribution with increasing time-lapse between images. A change in the accuracy of a recognition system can show up as a change in the authentic distribution, the impostor distribution, or both. The ‘impostor distribution’ measures that distribution between images of two different eyes. The ‘authentic distribution’ is the distribution of match values between two images of the same eye. Two parameters describe the accuracy of iris recognition systems. 4, 5 These results suggest that iris recognition systems need to implement some provision for re-enrollment. However, both our research group and another group recently published results showing that template aging does occur in iris recognition. While template aging is known to occur for biometrics that use the face and fingerprints, researchers have long believed that iris recognition was immune to this effect. ‘Template aging’ refers to increases in error rate over time between the original image (the ‘enrollment image’) and later images taken for identification (the ‘recognition image’). Images of the same iris from 2008 (above) and 2011 (below), acquired at University of Notre Dame using an LG 4000 iris sensor.
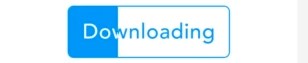